A new approach to the detection of machine-generated texts
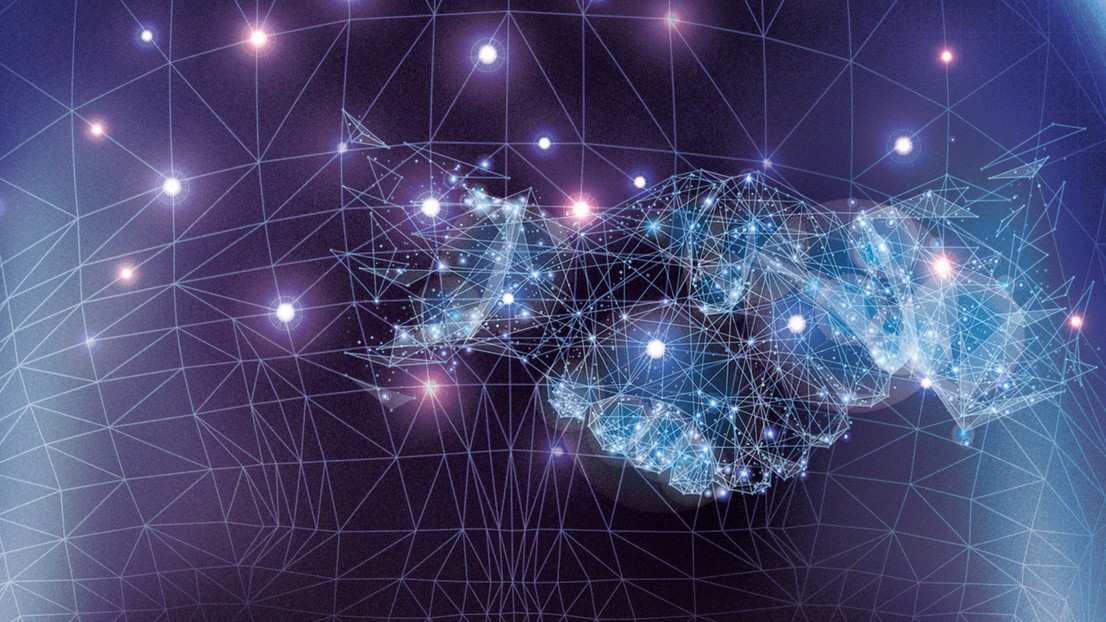
© 2021 Cyber-Defence Campus
Under the initiative of the armasuisse - Cyber-Defence Campus, a team of EPFL scientists, including CYD Distinguished Postdoctoral Fellow Andrei Kucharavy in the Distributed Computing Lab, have investigated a new approach to the detection of machine-generated texts. Machine-generated texts are a useful tool that can be used to our benefit – for instance to rapidly summarize large complex documents – such as for weather forecast or medical records. However, they can also be used to impersonate real people on the internet and to conduct disinformation and harassment campaigns, on and off social media.
In 2019, an internal Facebook report indicated that they were removing 3.2 billion fake accounts every six months. Basically, the entire world population worth of accounts every year. The scale of the misinformation and disinformation campaigns on the social media is hard to understate. They have not only the potential to interfere and derail legitimate public debate, but can also be used for other nefarious purposes such as knocking out the electricity grid, endangering critical infrastructure.
This makes the detection and removal of the impersonating accounts both critical and timely to cyber-defence. However, over the last two years, the operators of fake account networks have improved at avoiding detection, for example by generating realistic unique pictures for their accounts and generating back-stories to make the accounts look more legitimate.
These new avoidance techniques are majorly fueled by generative learning. On one hand, the deepfakes – photorealistic images generated by a type of AI called Generative Adversarial Networks (GANs), such as ThisPersonDoesNotExist.com – allowed them to create unique and attractive image profiles. On the other, AI-generated text provided by a type of AI called Transformers (no, not the Michael Bay ones) allowed them to create realistic-looking biographies and slowly accumulate posts including in languages they themselves do not speak - making them look like real people .
While the deepfakes – both for images and texts – that are available to the wide public still possess artifacts that render them detectable, the current generation of AIs restricted to researchers and large corporations, such as Google’s BigGAN or OpenAI’s GPT-3, are virtually undetectable. The GPT-3 in particular is concerning, because not only it is able to generate content that is indistinguishable from human-written ones, but this content is also highly engaging, reaching the top of meta-aggregation websites such as HackerNews.
One of the concerning developments is that tools developed to detect those generative models can actually be used in order to improve evasion from them. In fact, the cat-and-mouse game between a generative model and a model trying to tell the real images from the fake ones is exactly how the GANs are trained.
In order to investigate the feasibility of such a development, Kevin Blin and Andrei Kucharavy have investigated whether it would be possible to apply the GAN training model to the Transformer architectures directly, by choosing a task and architecture where a synergy would be expected.
Fortunately the results were negative – even in the best conditions, the combination of GANs and Transformers was struggling to train itself and was collapsing to producing gibberish. While it is still unclear if the combination of the two training models is impossible in practice for sufficiently large transformers (and hence well-performing – the two are linked together), this work shows that it is at least non -trivial and would require further research before it could pose a threat.
Overall, this work made it clear that scaling up social engineering attacks by combining these two approaches is not a simple task. Which is of course good news: attackers will have to find another way, at least for today.
The CYD Fellowships are supported by armasuisse Science and Technology.