A new AI approach to protein design
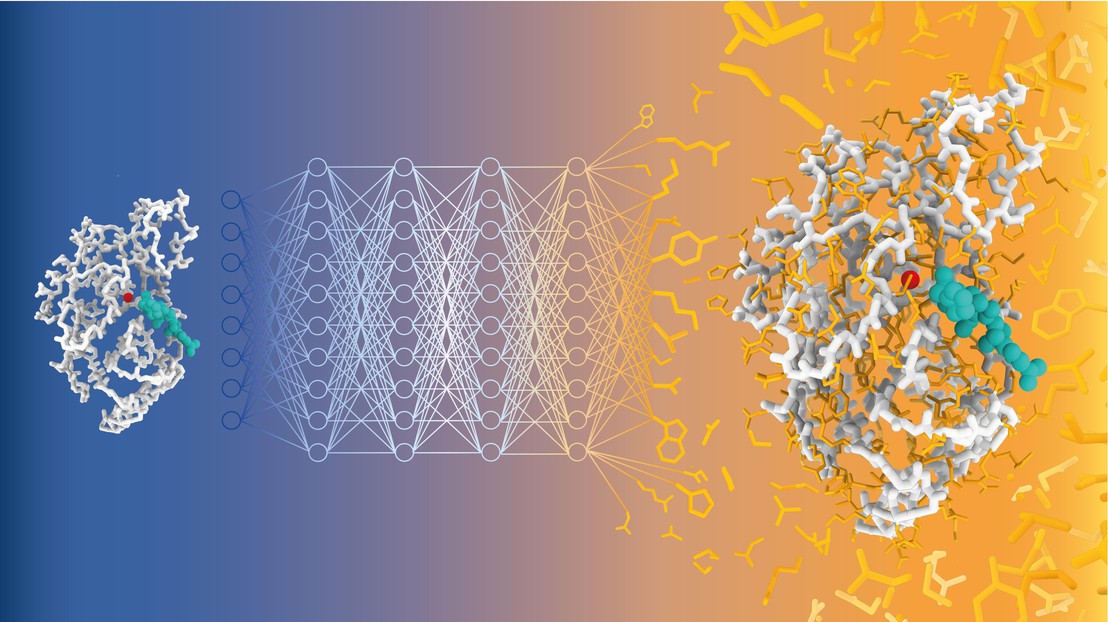
See text for caption. Credit: Alexandra Banbanaste (EPFL)
EPFL researchers have developed a novel AI-driven model designed to predict protein sequences from backbone scaffolds, incorporating complex molecular environments. It promises significant advancements in protein engineering and applications across various fields, including medicine and biotechnology.
Designing proteins that can perform specific functions involves understanding and manipulating their sequences and structures. This task is crucial for developing targeted treatments for diseases and creating enzymes for industrial applications.
One of the grand challenges in protein engineering is designing proteins de novo, meaning from scratch, to tailor their properties for specific tasks. This has profound implications for biology, medicine, and materials science. For instance, engineered proteins can target diseases with high precision, offering a competitive alternative to traditional small molecule-based drugs.
Additionally, custom-designed enzymes, which act as natural catalysts, can facilitate rare or nonexistent reactions in nature. This capability is particularly valuable in the pharmaceutical industry for synthesizing complex drug molecules and in environmental technology for breaking down pollutants or plastics more efficiently.
A team of scientists led by Matteo Dal Peraro at EPFL has now developed CARBonAra (Context-aware Amino acid Recovery from Backbone Atoms and heteroatoms), an AI-driven model that can predict protein sequences, but by taking into account the restraints imposed by different molecular environments – a unique accomplishment. CARBonAra is trained on a dataset of approximately 370,000 subunits, with an additional 100,000 for validation and 70,000 for testing from the Protein Data Bank (PDB).
CARBonAra builds on the architecture of the Protein Structure Transformer (PeSTo) framework – also developed by Lucien Krapp in Dal Peraro’s group. It uses geometric transformers, which are deep learning models that process spatial relationships between points, such as atomic coordinates, to learn and predict complex structures.
CARBonAra can predict amino acid sequences from backbone scaffolds, the structural frameworks of protein molecules. However, one of CARBonAra’s standout features is its context awareness, which is especially demonstrated in how it improves sequence recovery rates – the percentage of correct amino acids predicted at each position in a protein sequence compared to a known reference sequence.
CARBonAra significantly improved recovery rates when it includes molecular “contexts”, such as protein interfaces with other proteins, nucleic acids, lipid or ions. “This is because the model is trained with all sort of molecules and relies only on atomic coordinates, thus that it can handle not only proteins,” explains Dal Peraro. This feature in turn enhances the model's predictive power and applicability in real-life, complex biological systems.
The model does not perform well only in synthetic benchmarks but was experimentally validated. The researchers used CARBonAra to design new variants of the TEM-1 β-lactamase enzyme, which is involved in the development of antimicrobial resistance. Some of the predicted sequences, differing by approximatively 50% from the wild-type sequence, were folded correctly and preserve some catalytical activity at high temperatures, when the wild-type enzyme is already inactive.
The flexibility and accuracy of CARBonAra open new avenues for protein engineering. Its ability to take into account complex molecular environments makes it a valuable tool for designing proteins with specific functions, enhancing future drug discovery campaigns. In addition, CARBonAra's success in enzyme engineering demonstrates its potential for industrial applications and scientific research.
Swiss National Science Foundation (SNSF)
Swiss National Supercomputing Centre (CSCS)
Lucien F. Krapp, Fernando A. Meireles, Luciano A. Abriata, Jean Devillard, Sarah Vacle, Maria J. Marcaida, Matteo Dal Peraro. Context-aware geometric deep learning for protein sequence design. Nature Communications 25 July 2024. DOI: 10.1038/s41467-024-50571-y