2023 INFORMS Optimization Society Award for Wouter Jongeneel
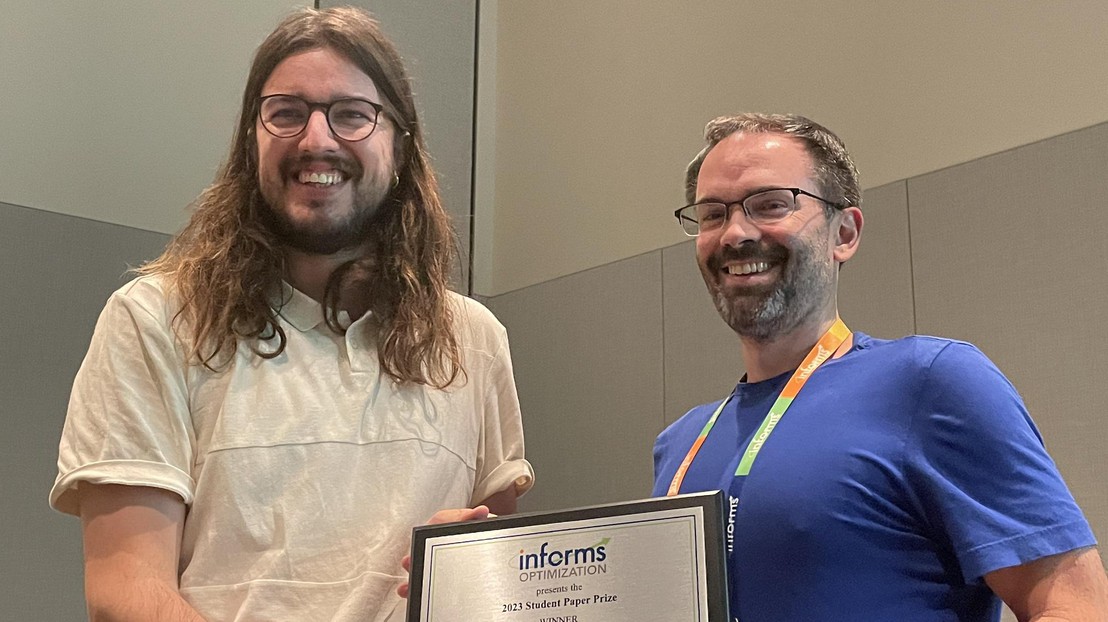
Wouter Jongeneel © 2023 EPFL
At the INFORMS Annual Meeting 2023 Wouter Jongeneel, PhD student of Daniel Kuhn, received the INFORMS Optimization Society Best Student Paper Award for his paper "Small Errors in Random Zeroth-Order Optimization are Imaginary" (co-authored with Man-Chung Yue and Daniel Kuhn).
With over 12,500 members from around the globe, INFORMS is the leading international association for professionals in operations research, analytics, management science, economics, behavioral science, statistics, artificial intelligence, data science, applied mathematics, and other relevant fields. The INFORMS Optimization Society Student Paper Prize, established in 2006 and administered by the INFORMS Optimization Society, is awarded annually to one (or more) students for the most outstanding paper in optimization that is submitted to and received, or published in a refereed professional journal within the three calendar years.
About the paper
The paper proposes a novel approach to zeroth-order optimization for solving continuous minimization problems and opens up new possibilities for numerically stable algorithms. A zeroth-order algorithm is derivative-free as it only makes use of function evaluations. Such methods are needed in simulation-based optimization, minimax optimization, reinforcement learning, etc. and typically belong to one of three classes: direct search methods, model-based methods, and randomized methods. Randomized methods have received much attention due to a recent paper by Nesterov and Spokoiny; the main idea is to choose a direction randomly, then decide how far to move along the direction based on a one-dimensional derivative estimate. Finite-difference-based derivative estimates suffer from numerical cancellation errors and cannot easily be used to achieve high precision. The approach in the paper by Jongeneel et al. is new and very interesting: It extends the domain of the objective function (assumed to be real-analytic) to a complex domain, then uses ideas from complex analysis to get a derivative estimate with a single function evaluation that is not subject to numerical cancellation. The proposed algorithm is shown to have approximation and convergence guarantees similar to multi-point estimators thereby combining the benefits of multi-point and single-point approaches. Convergence of a zeroth-order algorithm that employs these derivative estimates is proved in convex, strongly convex, and nonconvex settings. Numerical experiments show that the approach outperforms methods with comparable properties. The authors show how to apply their method to some problems where function evaluations come from simulations with PDEs. This is impressive. Overall, the paper addresses a fairly general problem and provides an elegant and surprising solution that has the potential to be widely applicable.
Small errors in random zeroth-order optimization are imaginary. W. Jongeneel, M.-C. Yue & D. Kuhn