When the neuroprosthetics learn from the patient
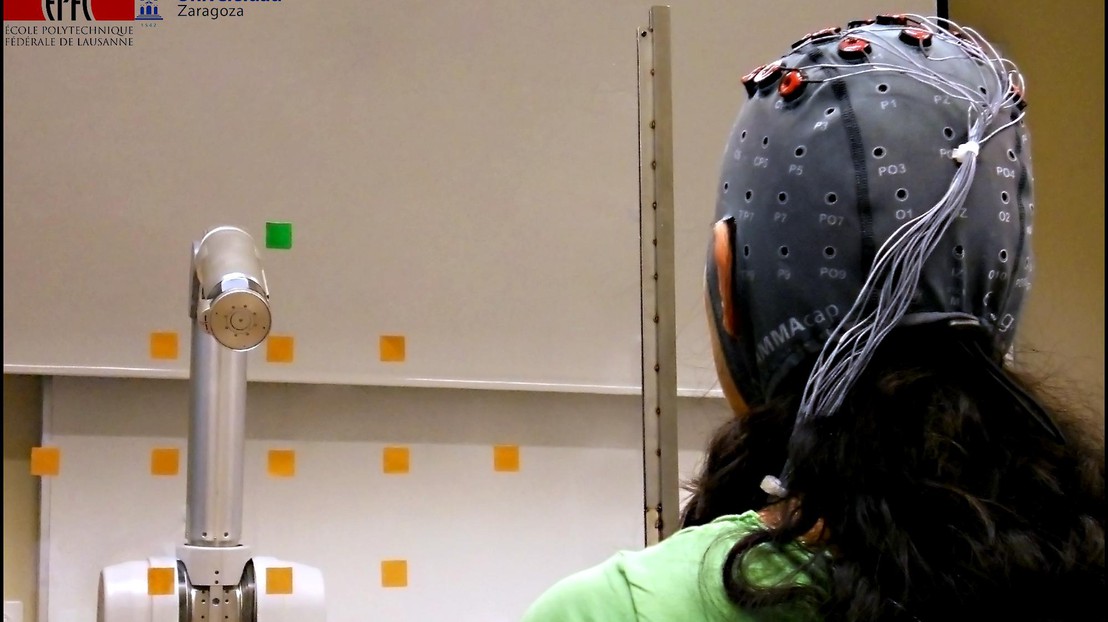
© 2015 EPFL
While it takes a long time to learn to control neuroprostheses, José del R. Millán's research, published in Nature Scientific Reports, will enable the creation of a new generation of self-learning and easy to use devices.
Brain-Machine Interfaces (BMI) are a great hope for thousands of patients affected by the most various motor impairments, notably paralysis. Patients fitted with such equipment can control artificial limbs through electrodes connected to their brains. In an article published today in Nature Scientific Reports, José Millán, holder of the Defitech Chair in Brain-Machine Interface, describes the application of an innovative technology that may allow the development of a new generation of BMIs.
Most BMI operate by interpreting variations in the electrical activity of the brain, in particular through an electroencephalogram. To be effective, such a method requires significant training on the patient's side. They must succeed in communicating the desired information (eg, "extend left arm") by modulating their brain activity. The results are encouraging but come up against two limits no matter how the brain electrical signals are recorded -invasively or not. Patients must spend a lot of time to learn to use their neuroprosthesis and despite this training, they are not able to perform some complex movements.
An "error signal"
When missing a step, the brain emits an electrical signal signifying the failure of the action. This signal is called Error-related potential (ErrP). José Millán, whose seminal work in the field of BMI was noted by the journal Science, uses this signal to develop a new generation of neuroprostheses. "With ErrPs, it is the machine itself that will learn to make the right movements." The EPFL professor calls this innovation a "paradigm shift."
The detection of this "error signal" allowed José Millán's team to create a new generation of neuroprostheses. They are capable of learning the proper movements based on ErrP. For example, if we fail to grasp a glass of water placed in front of us, the neuroprosthesis will understand that the action was unsuccessful and the next movements will change accordingly until the desired result is achieved. The machine knows that the goal is reached when the actions performed no longer generate an ErrP.
The paradigm shift lies in the use of these signals to relieve the subject from the tedious task of learning. This approach could be the source of a new generation of intelligent prostheses, able to learn a wide range of movements. Indeed, with the support of the ErrP decoder, it is theoretically possible to learn and master quickly enough a multitude of motor movements, even the most complex ones.
25 minutes to train its decoder
The twelve subjects of the experiment were first asked to train their prosthesis to detect ErrP. Equipped with an electrode headset, they observed the machine, programmed to fail in 20% of cases, performing 350 separate movements. The setting of the ErrP detector lasted on average 25 minutes. Once this first step was completed, the subjects performed three experiments to evaluate the effectiveness of this new approach. In the last one, they were asked to identify a specific target using a robotic arm placed two meters away. In all three experiments, the neuroprosthesis demonstrated interesting learning capabilities by continuously adapting its actions and enhancing its precision.
Indeed, the artificial arm stores the correct movements and builds an increasing repertoire of appropriates motors actions. This capability could be particularly useful for people with neurodegenerative diseases, allowing them to compensate almost organically the loss of motor function. "According to our expectations, this new approach will become a key element of the next generation brain-machine interfaces that mimic the natural motor control. The prosthesis can function even if it does not have clear information about the target," concluded José Millán.
This research was conducted in partnership with the Department of Computer Systems Engineering Research Institute of Aragon, University of Zaragoza (Spain), including Luis Montesano, Javier Minguez. Besides Professor Millán, Iñaki Iturrate and Ricardo Chavarriaga, from the Center for Neuroprosthetics and the Institute of Bioengineering at EPFL, also participated in this project.