Navigating a Molecular Database, with Machine Learning
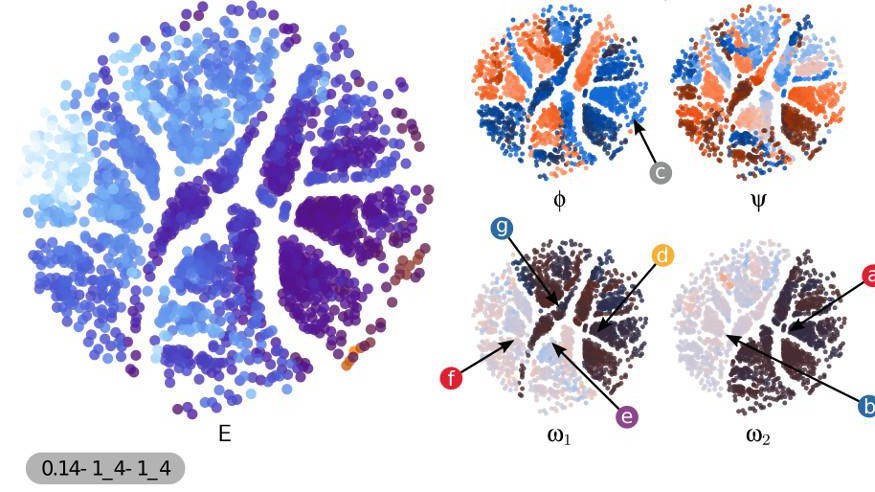
Maps representing the metastable conformers of Lysine dipeptide, colored according to molecular properties © COSMO/EPFL 2017
High-throughput computational materials design promises to greatly accelerate the process of discovering new and better materials. Machine learning can help visualizing and rationalizing the structure-property relations between structures in the large databases that result from automated materials searches.
The large databases of structures and properties that result from computational searches of materials, as well as the agglomeration of data of heterogeneous provenance leads to considerable challenges when it comes to navigating the database, representing its structure at a glance, understanding structure–property relations, eliminating duplicates and identifying inconsistencies. A recent publication from the laboratory of Computational Science and Modelling, funded by the National Competence Center in Research MARVEL, demonstrate the different ways machine-learning techniques can be used to assist materials scientists and chemists perform these taks.